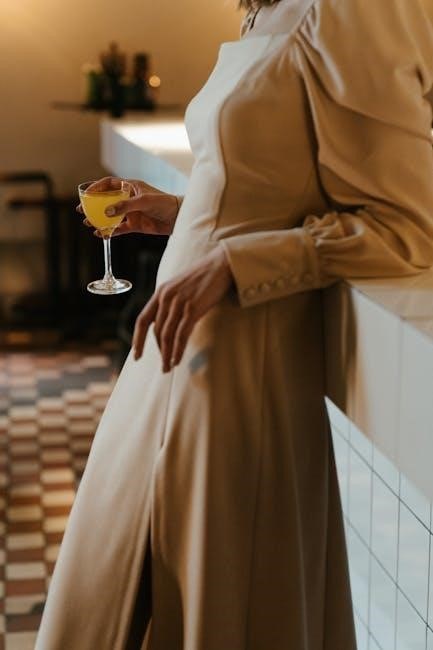
lean analytics alistair croll pdf
Lean Analytics, co-authored by Alistair Croll and Benjamin Yoskovitz, provides a data-driven approach to building startups faster. It focuses on using metrics to guide decision-making and growth.
1.1 What is Lean Analytics?
Lean Analytics is a framework by Alistair Croll and Benjamin Yoskovitz that helps startups use data to build faster. It focuses on tracking key metrics to guide decision-making and growth. This approach emphasizes validating ideas, finding the right customers, and determining what to build and monetize, ensuring data-driven decisions from inception onward.
1.2 The Role of Data in Building Startups Faster
Data plays a crucial role in accelerating startup growth by enabling informed decision-making. Lean Analytics emphasizes using metrics to validate ideas, identify target customers, and guide product development. By leveraging data, startups can prioritize features, measure progress, and optimize strategies, ultimately reducing uncertainty and fostering scalable growth.
About the Author: Alistair Croll
Alistair Croll is an entrepreneur, author, and public speaker known for co-authoring Lean Analytics. His work emphasizes data-driven decision-making to build successful startups.
2.1 Biography of Alistair Croll
Alistair Croll is a renowned entrepreneur, author, and public speaker. He co-founded several startups and has written extensively on entrepreneurship and analytics. His expertise in data-driven decision-making led to the creation of Lean Analytics, a guide for startups. Alistair is also a sought-after speaker, sharing insights on innovation and metrics. His work focuses on helping businesses grow efficiently using data.
2.2 His Contributions to Lean Analytics
Alistair Croll’s contributions to Lean Analytics are significant. He co-authored the book, which simplifies complex analytics for startups. His framework emphasizes tracking essential metrics to drive growth and validate ideas. Alistair’s approach helps entrepreneurs prioritize data, enabling smarter decisions and faster iteration. His work bridges the gap between data and actionable insights, making analytics accessible for businesses at all stages.
Key Concepts in Lean Analytics
Lean Analytics focuses on metrics that matter, data-driven decision-making, and the Lean Startup methodology. It emphasizes tracking the right metrics to validate ideas and drive growth efficiently.
3.1 Metrics That Matter
In Lean Analytics, Alistair Croll emphasizes identifying and prioritizing key metrics that directly impact business goals. These metrics vary by startup stage, helping entrepreneurs focus on what truly drives growth and validation. By concentrating on actionable data, startups can make informed decisions, optimize resources, and align efforts with customer needs and market demands effectively.
3.2 Data-Driven Decision Making
Data-driven decision making is central to Lean Analytics, enabling startups to validate ideas, reduce uncertainty, and optimize processes. By leveraging actionable metrics, entrepreneurs can make informed choices, ensuring alignment with customer needs and market demands. This approach fosters a culture of continuous improvement, helping businesses refine their offerings and scale effectively while maintaining focus on measurable outcomes.
3.3 The Lean Startup Methodology
The Lean Startup Methodology, intertwined with Lean Analytics, emphasizes rapid product development and iterative learning. It advocates for building, measuring, and learning in a continuous cycle, ensuring startups efficiently allocate resources. By focusing on validated learning, entrepreneurs minimize waste and maximize value creation, aligning their efforts with customer needs and market demands for sustainable growth and innovation.
The Importance of Lean Analytics in Startups
Lean Analytics is vital for startups as it enables data-driven decision-making, ensuring resources are allocated efficiently. It helps validate ideas quickly, reducing risks and fostering sustainable growth.
4.1 Why Lean Analytics is Essential for Success
Lean Analytics is crucial for startups as it prioritizes actionable data, enabling informed decisions. By focusing on key metrics, startups can validate ideas, identify customer needs, and optimize resources. This approach minimizes uncertainty, accelerates growth, and ensures alignment with business goals, making it indispensable for achieving long-term success in competitive markets.
4.2 Solving Common Startup Problems with Data
Lean Analytics tackles startup challenges by leveraging data to validate assumptions. It helps identify real problems, find the right customers, and refine products. By focusing on metrics like customer acquisition and retention, startups can address pain points effectively, ensuring scalability and sustainable growth, ultimately turning data into actionable insights for success.
Structure of the Book “Lean Analytics”
Lean Analytics is divided into four parts, covering metrics, decision-making, and the lean startup methodology. It offers a comprehensive, hands-on guide to building startups with data-driven insights.
5.1 Overview of the Four Parts
The book is structured into four parts, each addressing key aspects of lean analytics. The first part introduces foundational metrics and principles, while the second delves into decision-making processes. The third part explores the lean startup methodology, and the final section provides practical tools for implementation. This structure ensures a comprehensive understanding of data-driven startup growth.
5.2 Key Takeaways from Each Section
Each section of “Lean Analytics” offers actionable insights. The first part emphasizes identifying the right metrics for startups. The second highlights using data to make informed decisions. The third focuses on aligning analytics with the lean startup methodology. The final part provides practical tools and frameworks for implementing lean analytics effectively, ensuring readers can apply these concepts to their own businesses.
Case Studies and Real-World Applications
Real-world examples demonstrate how startups successfully applied lean analytics to validate ideas, measure progress, and scale efficiently, offering valuable lessons for entrepreneurs and businesses.
6.1 Successful Startups Using Lean Analytics
Startups like Dropbox, Airbnb, and LinkedIn have successfully applied lean analytics to validate ideas, measure progress, and scale efficiently. These companies leveraged data to identify key metrics, optimize customer acquisition, and refine their business models. By focusing on actionable insights, they accelerated growth and achieved sustainable success, demonstrating the practical value of lean analytics in real-world scenarios.
6.2 Lessons Learned from Implementing Lean Analytics
Implementing lean analytics teaches startups to prioritize one key metric at each stage, ensuring focus and clarity. It emphasizes rapid experimentation, customer feedback, and iterative improvement. Businesses learn to avoid vanity metrics and instead track actionable data that drives growth. By fostering a culture of continuous learning, lean analytics helps startups adapt quickly to market changes and build sustainable growth strategies effectively.
How to Download the Lean Analytics PDF
The Lean Analytics PDF by Alistair Croll can be downloaded from O’Reilly or online archives. Scan the QR code or visit the provided link to access it.
7.1 Sources for the PDF Version
The Lean Analytics PDF by Alistair Croll and Benjamin Yoskovitz is available from O’Reilly, online archives, and open-source collections. Scan the provided QR code or visit linked platforms for direct access. Ensure authenticity by downloading from trusted sources to avoid unauthorized versions.
7.2 Tips for Reading and Applying the Content
Start with the introduction to grasp core concepts. Prioritize metrics aligning with your goals. Apply data-driven decisions incrementally. Test ideas early and often. Leverage case studies for practical insights. Focus on continuous learning and iteration to maximize impact. Use the frameworks provided to tailor strategies to your business needs. Regularly review and adapt your approach as your startup evolves.
Implementing Lean Analytics in Your Business
Start with a clear plan, identify key metrics, and use tools to track progress. Regularly test hypotheses and iterate based on data to align with business goals.
8.1 Step-by-Step Guide to Getting Started
Begin by defining your startup’s mission and identifying the problem you aim to solve. Next, validate your idea through customer interviews and surveys to ensure market demand. Develop a minimum viable product (MVP) to test your hypothesis and gather initial feedback. Implement analytics tools to track user behavior and key metrics. Use the data to refine your product, prioritize features, and optimize your business model. Continuously iterate and experiment to improve customer acquisition and retention. By following these steps, you can effectively integrate lean analytics into your business strategy and drive sustainable growth.
8.2 Tools and Resources for Effective Implementation
Utilize tools like Google Analytics for web tracking, Mixpanel for user retention insights, and Hotjar for understanding user behavior. Segment.io helps integrate data sources, while Tableau enables visualization. The Lean Analytics PDF by Alistair Croll offers practical guidance. Additionally, online courses on platforms like Coursera and resources from Lean Startup Co. can enhance your implementation skills and ensure data-driven decision-making.
Criticisms and Limitations of Lean Analytics
Critics argue that Lean Analytics may overemphasize quantitative data, potentially neglecting qualitative insights. Its focus on singular metrics can oversimplify complex business challenges, limiting holistic understanding.
9.1 Common Criticisms of the Approach
While Lean Analytics is highly valued, critics argue it may overemphasize quantitative data, potentially neglecting qualitative insights. Some suggest its focus on singular metrics can oversimplify complex business challenges, while others argue it may not fully account for market dynamics or innovation. These criticisms highlight the need for a balanced approach when applying Lean Analytics principles in practice.
9.2 Addressing Limitations in Practice
To address the limitations of Lean Analytics, businesses should combine quantitative insights with qualitative feedback. Integrating diverse data sources and iterative testing can help mitigate oversimplification. Additionally, balancing Lean Analytics with broader strategic frameworks ensures comprehensive decision-making. By adopting a holistic approach, organizations can maximize the benefits of Lean Analytics while addressing its potential shortcomings in dynamic market environments.
Common Metrics in Lean Analytics
Key metrics include Customer Acquisition Cost (CAC), Lifetime Value (LTV), retention rates, and churn rates. These metrics help validate ideas and guide product development efficiently.
10.1 Key Performance Indicators (KPIs) to Track
In Lean Analytics, Alistair Croll emphasizes tracking KPIs like Customer Acquisition Cost (CAC), Lifetime Value (LTV), retention rates, and churn rates. These metrics help validate ideas, optimize marketing, and improve customer satisfaction, ensuring sustainable growth. By focusing on actionable data, startups can make informed decisions and align their strategies with customer needs effectively.
10.2 How to Prioritize Metrics for Your Business
Alistair Croll advises prioritizing metrics based on business goals and customer lifecycle stages. Focus on metrics that indicate progress toward key objectives, such as user engagement or revenue growth. Use a data-driven framework to align metrics with strategic priorities, ensuring each KPI directly impacts business outcomes. This approach helps startups allocate resources effectively and measure success systematically.
The Future of Lean Analytics
The future of Lean Analytics lies in advanced tools and AI integration, enabling faster data-driven decisions. It will focus on real-time insights and simpler processes for startups.
11.1 Emerging Trends in Data-Driven Startups
Data-driven startups are embracing AI and machine learning for predictive analytics. Real-time decision-making tools and automated insights are becoming essential. Lean Analytics principles are evolving to integrate with modern technologies, enabling faster iteration and smarter scaling. Startups now focus on ethical data usage and transparency, ensuring sustainable growth. These trends highlight the growing importance of actionable data in building resilient businesses.
11.2 How Lean Analytics Will Evolve
Lean Analytics will evolve by integrating advanced AI and machine learning tools, enabling real-time data processing. The focus will shift to automating insights and simplifying complex datasets. As startups grow, Lean Analytics will adapt to handle larger-scale data while maintaining agility. Future iterations will emphasize ethical data practices and transparency, ensuring sustainable growth without compromising innovation or customer trust.
Lean Analytics offers a powerful framework for startups to leverage data effectively, enabling faster growth and informed decision-making. Alistair Croll’s insights empower entrepreneurs to build smarter businesses.
12.1 Summary of Lean Analytics Principles
Lean Analytics emphasizes using data to validate assumptions and guide decision-making. It focuses on identifying key metrics, prioritizing actionable insights, and iterating based on feedback. By aligning metrics with business goals, startups can optimize growth and efficiency, ensuring resources are used effectively. Alistair Croll’s approach underscores the importance of simplicity and actionable data in driving successful outcomes.
12.2 Final Thoughts on Implementing Lean Analytics
Implementing Lean Analytics requires a commitment to data-driven decision-making and continuous learning. By focusing on key metrics and iterating based on insights, businesses can achieve sustainable growth. Alistair Croll’s framework provides a clear roadmap for startups to leverage data effectively, ensuring they build products that meet market demands and drive long-term success.